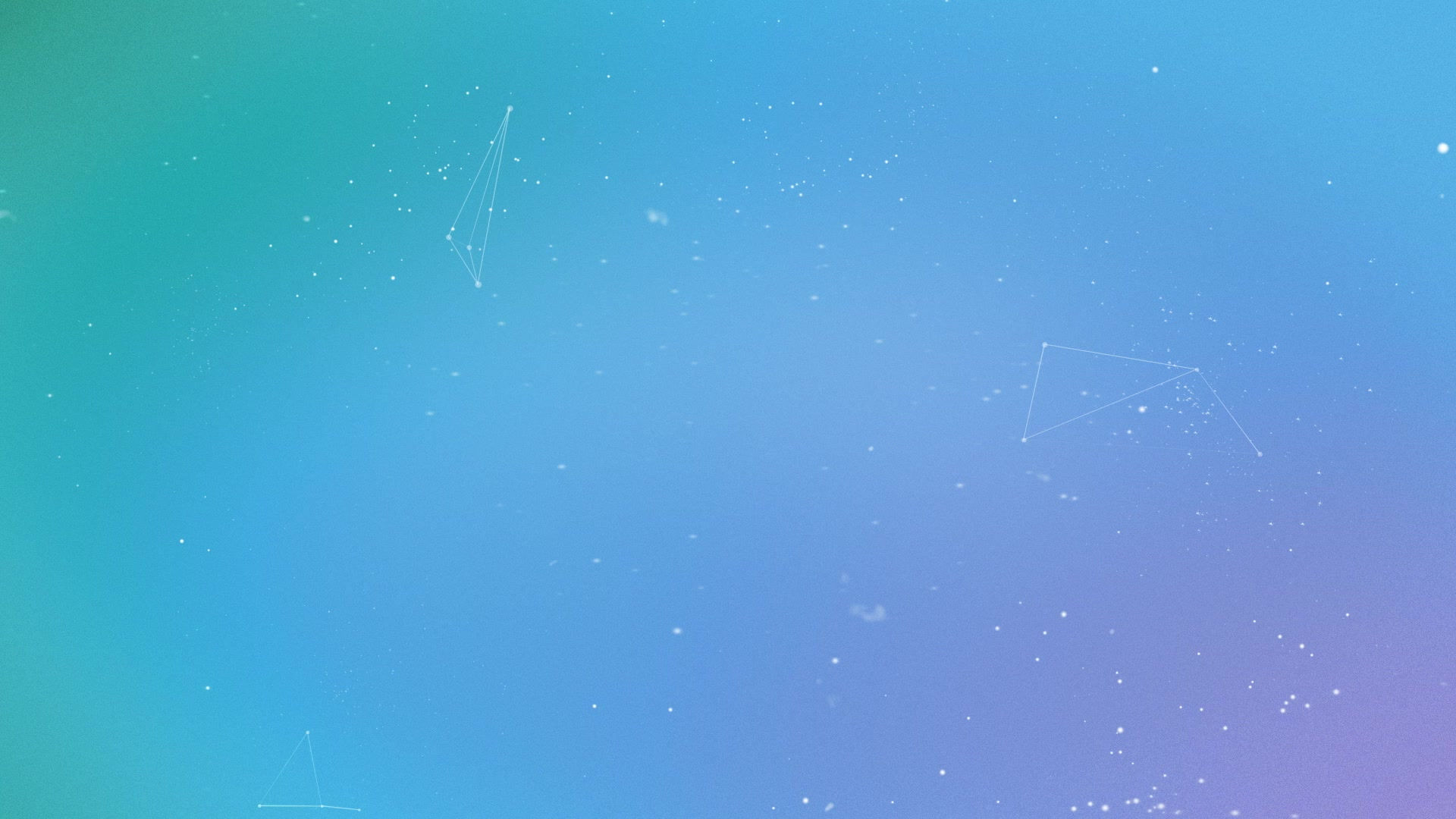
Monitoring seasonal maize phenology
Luciana Nieto, Dr. Raí Schwalbert, Dr. P. V. Vara Prasad,
Dr. Bradley J.S.C. Olson , Dr. Ignacio A. Ciampitti
Efficient and more accurate reporting of maize (Zea mays L.) phenology, crop condition, and progress is crucial for agronomists and policymakers. Integration of satellite imagery with machine learning models has shown a great potential to improve crop classification and provide in-season progress reports. However, the precision of the crop phenology classification needs to be substantially improved to transform data into actionable management for farmers and agronomists. An integrated approach of ground-truthing field data for maize crop phenology (2013-2018 seasons), satellite imagery (Landsat 8), and weather data was explored with the following objectives: i) model training and validation – find the best combination of spectral bands, vegetation indices (VIs), weather parameters, geolocation, and ground truth data, leading to a model with the highest accuracy across years at each season segment (step one) and ii) model testing – once the model has been selected, the performance for each phenology class was tested using unobserved data (hold-out cross-validation) (step two). The best model performance for classifying maize phenology was documented when VIs (NDVI, EVI, GCVI, NDWI, GVMI) and vapor pressure deficit (VPD) were used as input variables. This study supports the integration of field ground-truthing, satellite imagery, and weather data to classify maize crop phenology facilitating decision making, agricultural interventions, and providing foundational knowledge for the different actors of the agricultural chain.
​
​